
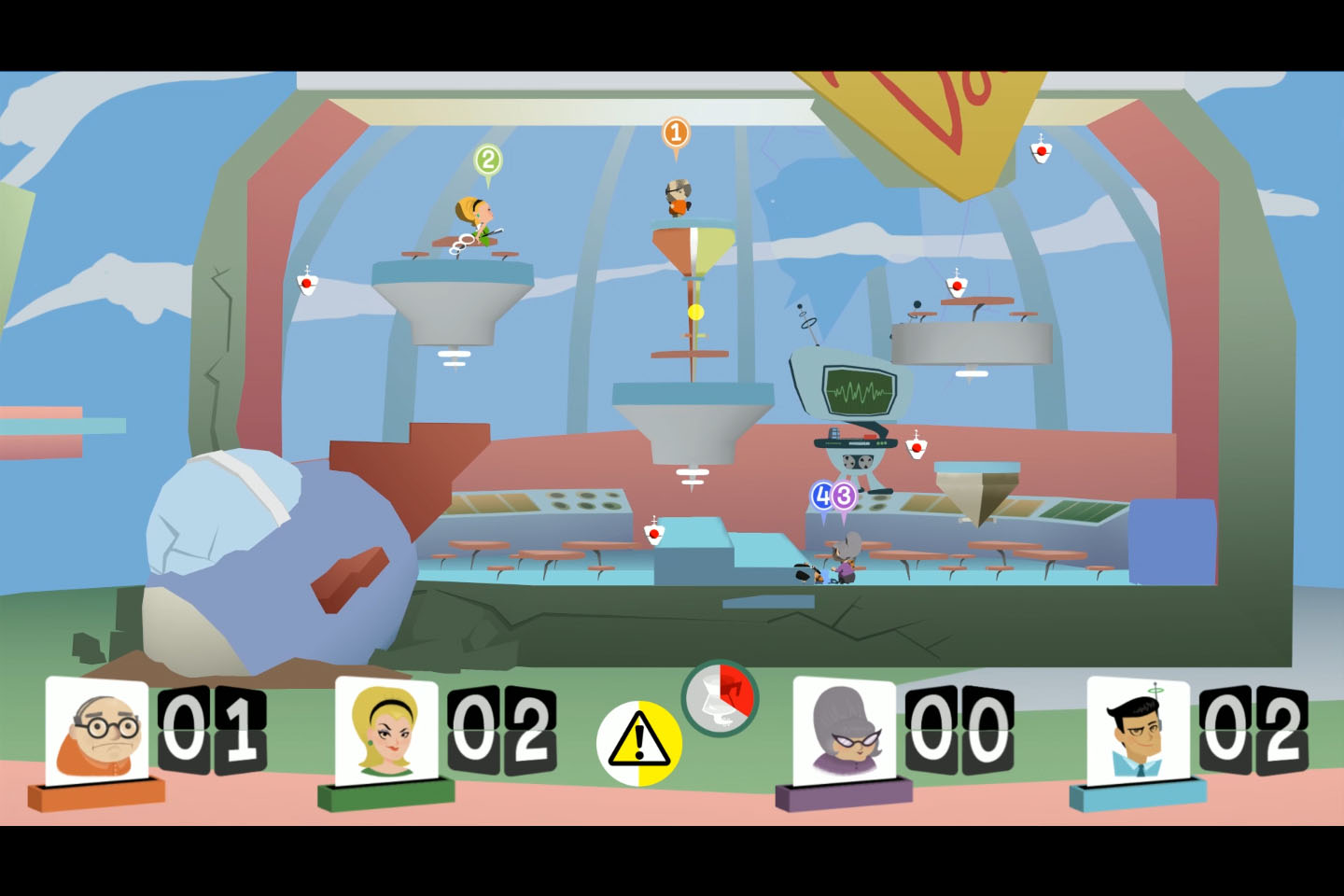
Taking those models as a baseline, they started pitting small variations of the models against themselves in a tournament-like fashion. First, it trained on millions of hours of Go gameplay from humans. The team of DeepMind didn’t give it any strategy. They made a large neural network model that only knew the rules of Go. It’s way harder and, therefore, a new challenge for AI to overcome. Think of it like this: multiply the number of chessboard configurations by the number of stars in the universe, then multiply the result by the number of stars in the universe again, and that is still factors lower than the number of board configurations for Go. If we compare chess with Go, chess might have more rules, but Go has more unique board configurations. Why Go?Īfter chess, Go was the next big logical game for the AI community.
#COFFEE BREAK GAMES MOVIE#
For more details, check out the (very entertaining!) movie on YouTube about this match and the events that led up to it. The general idea was that AI wouldn’t be able to beat a human player at Go for a long time, but due to neural networks, DeepMind was able to do this already in 2016! They beat the world champion of Go, Lee Sedol.
#COFFEE BREAK GAMES HOW TO#
It needed to understand the rules, figure out what the controls did, learn how to score points and develop a strategy, without any hardcoded information about the game itself.Īpplying this kind of research is not only fun, but it is also a step towards autonomous learning in real-world environments! The developers did not tell the models how Atari Breakout works, the model needed to learn the mechanics of the game only from visual information. These kinds of models can figure out the rules of the application on their own. What are the benefits?Īpplying AI to games is beneficial because it gives access to an environment that is easily controllable and understandable by the people implementing the model. It even learned that digging a tunnel through the side of the level, so the ball keeps bouncing on top of the blocks, is a good strategy. It learned through multiple iterations that the longer the ball was in play, the more blocks were hit and the higher the score would be. Then the reinforcement learning was trained on this data, with the goal to maximize the score that is on the screen. So, the convolutional neural network converted the pixels of the game into numerical values that can be understood by the reinforcement learning model. Almost all machine learning models need numerical values as input, but a screen capture is just a bunch of pixels. The DeepMind team captured the Atari Breakout screen while playing and then used the graphical information as input for a convolutional neural network (which is a type of model that can take in both images and video as input). Therefore, using Atari breakout in this research also provides insight on how to process visual information using machine learning. When teaching a model how to play these games, there has to be a way to capture what is happening on the screen, so the model can use that information to learn. In addition, when humans play Atari games, they play it on a screen with a controller. It’s a scoped first step in applying neural networks in combination with reinforcement learning. The model only needed to take into account the game screen and score and that was all. Why Atari breakout?Ītari Breakout is a minimal, contained application, with few variables.

They released a paper in 2013 and a short video in 2015 showing the AI playing. Using this, DeepMind was the first to create a model that beat the Atari game Breakout in 2013. Think of reinforcement learning as a computer model trying out different things, getting a reward when it does well, getting a penalty when it does bad, and learning from this interaction. Multiple articles were released on how neural networks outperformed state of the art algorithms.ĭeepMind, a company founded in 2010 and bought by Google in 2014, jumped on this technique by using deep learning and applying it to reinforcement learning. Let’s dive in!Ģ012 was a breakthrough year for neural networks and deep learning. In this chapter, we highlight three milestones where AI did use neural networks to solve their cases.
#COFFEE BREAK GAMES SERIES#
Welcome back to our blog series Coffee Break with AI! The previous blog went over chess, Jeopardy! and poker, and showed they were all solved by AI models that don’t use neural networks. Coffee Break with AI is brought to you by Elisio Quintino and Martijn Loos.
